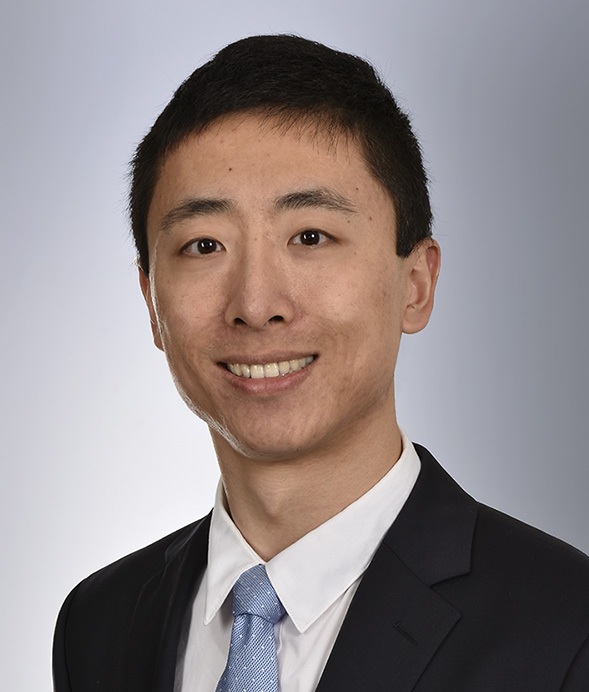
The Role of AI in Environmental Analyses
Oral Presentation
Prepared by R. Luo1, T. King2, W. Chau3, E. Cerda4, E. Parry5, T. Anumol6
1 - Agilent Technologies, Inc., 5301 Stevens Creek Blvd,, Santa Clara, California, 95051, United States
2 - Agilent Technologies, Inc., Wan Chai, Hennessy Rd, Hang Seng Wanchai Building, , 999077, Hong Kong
3 - Agilent Technologies Inc., Wan Chai, Hennessy Rd, Hang Seng Wanchai Building, , 999077, Hong Kong
4 - Agilent Technologies, Inc., 6705 Millcreek Dr, Mississauga, Ontario, L5N 8B3, Canada
5 - Agilent Technologies, Inc., 2850 Centerville Rd, Wilmington, Delaware, 19808, United States
6 - Agilent Technologies, Inc., 2850 Centerville Rd, DE, Wilmington, Delaware, 19808, United States
Contact Information: [email protected]; 470-981-6107
ABSTRACT
Environmental analysis of pesticides, PAHs, PCBs and other regulated compounds at trace level is challenging, even when advanced techniques, such as mass spectrometry, are utilized. Sample matrices can strongly impact on the background signal, increasing the difficulty of correct peak integration. Sophisticated sample preparation and cleanup steps might unintentionally introduce new contaminants into the samples or variety of different sample matrices can be a challenge too.
A machine learning (ML) architecture, designed for GC/MS data processing software allows continuous “learning” of lab-specific methods for various testing services. The plug-in tool operates first in passive mode, building a ML model based on a chemist’s current data analysis workflow. Data analysis results are continuously monitored and fed into a training pipeline to generate a model using a deep learning neural network. Once learnt, the tool replaces the manual tasks, including baseline correction, peak combining or splitting, and elimination of false positive or negative peaks.
A ML model for the phthalates analysis in consumer products was developed. Other models are in the training stage. In the case of phthalate analysis, isomeric compounds, such as diisononyl phthalate (DINP) and diisodecyl phthalate (DIDP), result in broad, irregularly shaped peaks usually requiring additional manual integration. The fully trained ML model can correctly integrate all phthalates investigated in this study, including DINP and DIDP. Retention time shift and change of qualifier ratio sometimes might occur during the GC/MS data acquisition, especially after the maintenance or column replacement. Both parameters were considered during the model training process. With the fully trained ML model, the quantifier ion as well as the corresponding qualifier ion(s) of each phthalate can be correctly integrated across the calibration range. The data analysis time was reduced to 1/5 to 1/4, when the manual integration step is replaced by the prediction of the fully trained ML model.
The GC/MS data processing plug-in tool with a continuously learning model provides reproducible and reliable results, drastically reduces number of manual integrations, therefore reduces the overall data analysis time.