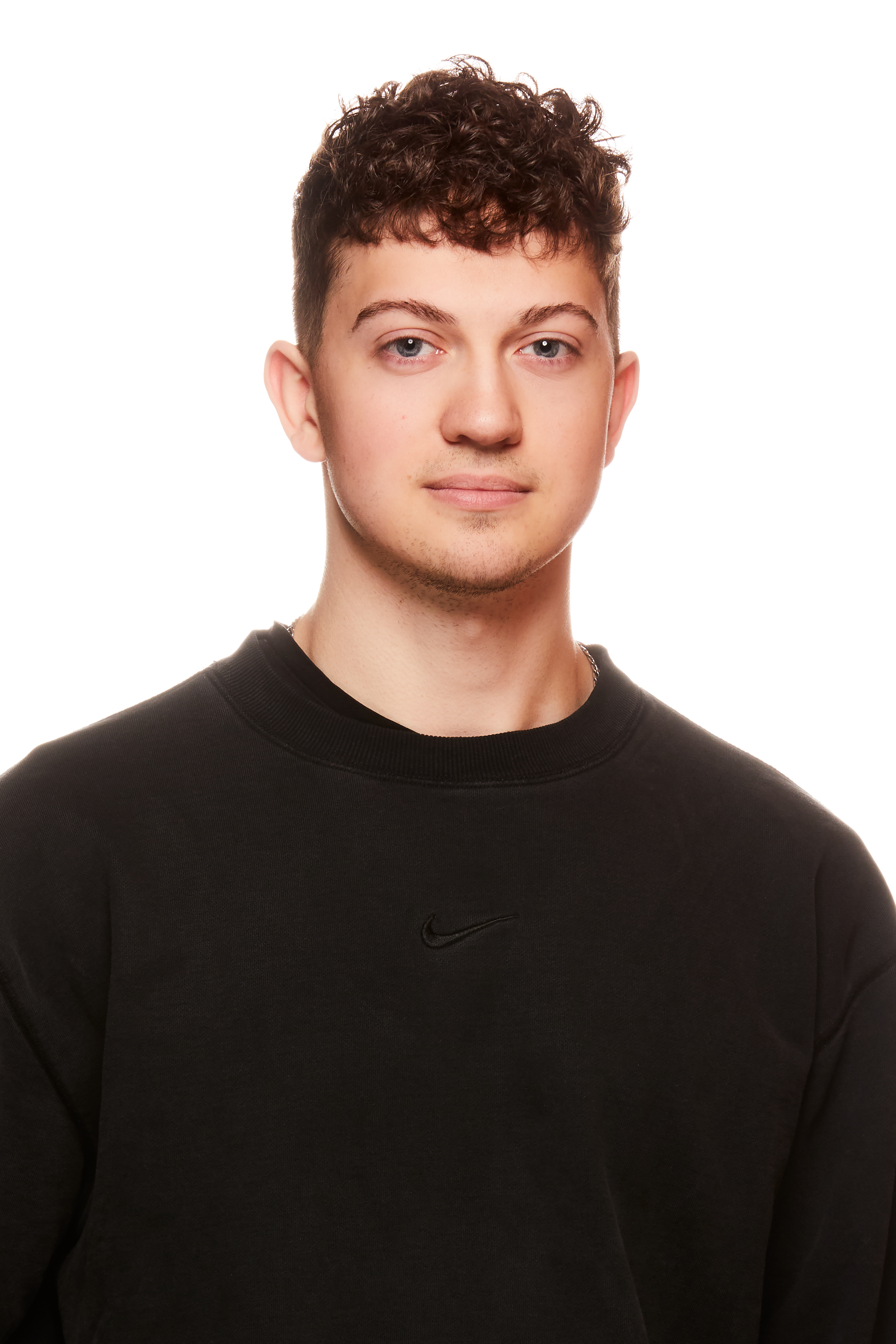
The Role of AI in Environmental Analyses
Oral Presentation
Presented by N. Chambers
Prepared by D. Hodarenko, D. Gamiles, J. Flint, K. Grah
Argos Scientific, Inc., 4600 NW Camas Meadows Dr, Suite 100, Camas, Washington, 98607, United States
Contact Information: [email protected]; 360-843-6359
ABSTRACT
With the growing adoption of community and fence-line air monitoring initiatives nationwide, the effective use of collected data becomes paramount. A principal application of this data involves identifying emission sources and predicting downwind community exposure. Large datasets from open-source, real-time websites, encompassing both open-path systems and point monitors within a region, offer a means to evaluate potential health impacts on local communities. A cutting-edge method involves applying machine learning to analyze data from multiple sources, thus identifying correlations between long-path and point monitoring systems across a wide community area. This method utilizes sophisticated statistical and data processing algorithms for the near-real-time pinpointing of emission sources. The derived data is crucial for assessing the health effects of fugitive emissions from complex industrial sources.
The data is showcased to illustrate the integration of reports from open-path UV air monitoring systems with point sampling data and an array of analyzers to identify fugitive emissions. When combined, these datasets assist in estimating the emission plume characteristics from local pollution sources. This information, alongside meteorological data, is then employed to gauge the downwind impact of these sources on at-risk communities. Furthermore, identifying the sources of emissions enables the implementation of subsequent mitigation measures.