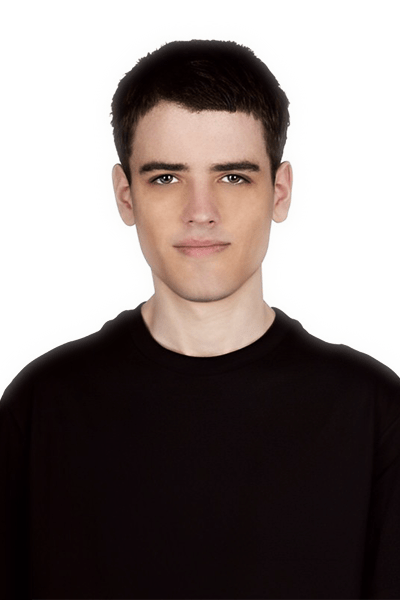
The Role of AI in Environmental Analyses
Oral Presentation
Presented by R. Berge
Prepared by H. Gebhart1, N. Chambers1, S. Lapin2, J. Flint1, R. Berge1
1 - Argos Scientific, Inc., 4600 NW Camas Meadows Dr, Suite 100, Camas, Washington, 98607, United States
2 - Washington State University, 915 N Broadway, Everett, Washington, 98201, United States
Contact Information: [email protected]; 503-519-2222
ABSTRACT
The increasing need for precise air quality information underlines the importance of improving environmental monitoring techniques. This abstract introduces a cutting-edge approach that utilizes artificial intelligence (AI) and machine learning (ML) to harness ambient quality assurance (QA) pollutants – such as ozone, methane, and nitrogen oxide – as benchmarks for the validation of monitoring system performance. Unlike traditional methods that depend on periodic manual checks and scheduled maintenance, this approach integrates AI and ML to address data quality issues in real-time.
The methodology involves the use of ML algorithms to analyze real-time data from ambient QA pollutants collected by reliable sources, including ambient air monitoring stations operated by regulatory agencies. This data is then compared with readings from internal sensors to validate their accuracy and ensure the monitoring equipment's optimal performance. By leveraging credible external environmental data, the system provides a robust validation mechanism for ambient QA pollutants measurements.
Additionally, the integration of AI into the system employs historical maintenance data as a basis for training, identifying patterns that inform calibration and predictive maintenance schedules. This not only ensures the reliability of the monitoring systems but also enables the early identification of potential calibration drifts or equipment failures through continuous analysis of discrepancies between internal and external data.
The application of this innovative approach to fence-line air monitoring networks in regions such as San Francisco and Los Angeles exemplify its effectiveness in enhancing the quality of air monitoring systems mandated by regulatory agencies. How this technology empowers communities and regulatory bodies to assess and improve the accuracy and reliability of environmental monitoring systems will be presented.