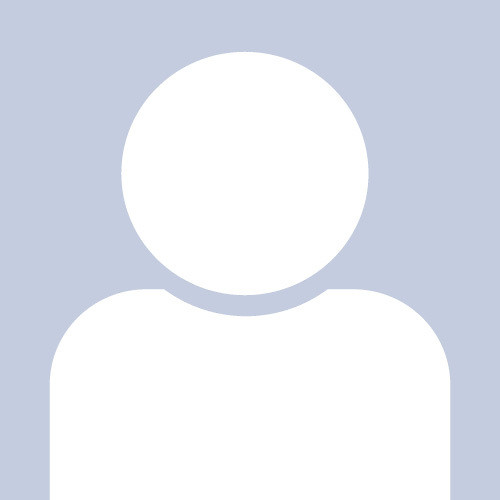
Laboratory Informatics
Oral Presentation
Presented by J. Westra
Prepared by N. Tintle1, J. Westra1, A. Best2, B. Krueger2, K. Vande Griend2, A. Slater3
1 - Superior Statistical Research, 1606 4th Ave SE, Sioux Center, IA, 51250, United States
2 - Aquora Research and Consulting, 6486 Castle Ave, Holland, MI, 49423, United States
3 - Aquora Research and Consulting, 6486 Castle Ave., Holland, MI, 49423, United States
Contact Information: [email protected]; 712-635-4811
ABSTRACT
At least 45 million people per year in the U.S. are directly impacted by health-based drinking water problems. Many of these water problems are the direct result of managerial negligence, inconsistent monitoring, and a lack of the ability to anticipate where problems may arise next. While the reasons for drinking water problems are complex, if we could anticipate where health-based drinking water problems were to occur in the future, it could have an immediate and positive impact on tens of millions of Americans annually. Extensive data about water quality and the performance of municipal water systems already exists in large, disparate databases. We have developed tools that mine these existing databases of administrative violations, sub-threshold water-quality results and other data in order to accurately predict future drinking water crises. In a pilot application of our approach, we demonstrated that we could predict cities that were on the cusp of health-based water crises. In particular, the Superior Statistical Research (SSR) next-generation algorithm – InsightSSR – prospectively predicted 10 communities across Iowa and Michigan which, when we visited and conducted wide-ranging water tests, all showed elevated levels of predicted pollutants. Thus, we not only knew where problems would occur – but what the problems would be. Proof of our concept models in two states have positioned us for widespread (multi-state) database harmonization and improvement of the machine-learning/modelling approach.